This post is reviewing a paper "Server-Driven Video Streaming for Deep Learning Inference" by Kuntai Du, and Ahsan Pervaiz presented in SIGCOMM ’20, August 10–14, 2020, Virtual Event, USA.
Motivation
- Video analytics allows for aggressive video compression.
- In contrast, human viewer requires more high-quality videos.
- Video analytics enables aggressive compression on non-object pixels.
- Only the server-side DNN has sufficient info to guide efficient video compression or streaming.
Idea
Drive video streaming by the real-time feedback from the server-side DNN. ➡️ DNN-Driven Streaming(DNS)
Background
- Video analytics has become more pervasive. ex) Traffice camera, wild-life camera
- ➡️ Let's Scale-Out video analytics
- Depending on the inference hardware, there is big difference in the inference accuracy.
- MobileNet in local camera vs FsterRCNN-ResNet in GPU-equipped server
- The camera's local inference is inaccurate.
- ➡️ We need to stream the video to the server for accurate inference
Design goals of video streaming protocol
- Preserver high accuracy
- Save bandwidth
Previous works for saving bandwidth
- Camera-side heuristics
Camera filters out irrelevant components.
(-) Low accuracy: The camera-side heuristics may miss many objects which cannot be recovered later by the server DNN. - Video encoding informed by server DNN
Server chooses a video-codec configuration for the incoming video and stream it to camera. Camera encode the video with video encode configuration and stream back to the server.
(-) High bandwidth consumption: They pay too much bandwidth on non-object pixels
(-) They need several minutes to adapt to new content. They cannot react to real-time content change.
DNN-Driven Streaming: Iterative workflow
- Generate all regions that may contain objects
- Eliminate regions that overlap with high-confidence inference results
- Encode remaining regions in codec-friendly manner
➡️ DDS find regions where DNN is indecisive
- Camera sends low-quality video to server
- Server performs DNN inference and sends the inference results(low confidence area) to the camera
- Camera encode high quality video of low confidence area to the server
- Server performs DNN inference of low confidence area and update inference results
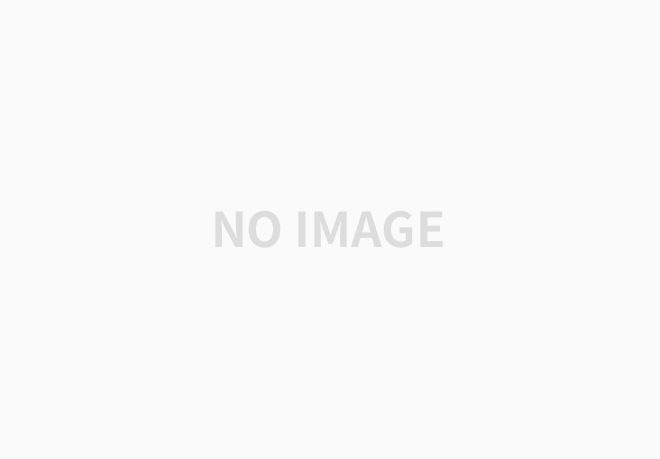
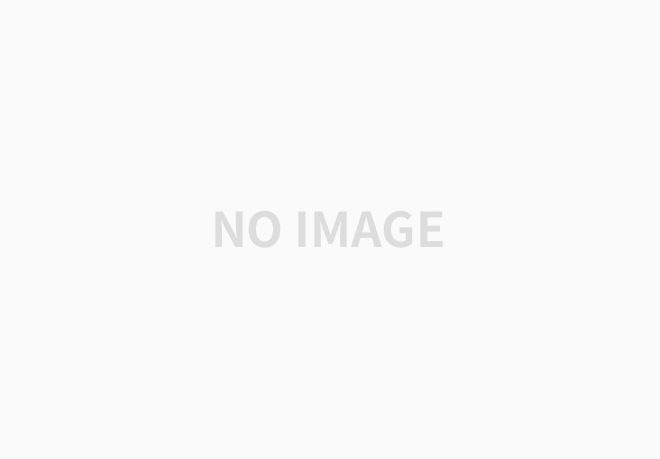
Experiment
- Dataset: 49 videos(Traffic camera, dacham, drone, and face videos)
- 3 tasks: Object detection, Semantic segmentation, Face recognition
Result
- DDS can save up to 50% bandwidth and achieve higher accuracy
- Bandwidth savings vary with videos and queries
- DDS saves about 50% streaming under various bandwidth
- The end-to-end delay of DDS is consistently lower than AWStream